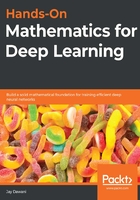
Random variables
Random variables are variables that have a probability distribution attached to them that determines the values each one can have. We view the random variable as a function, X: Ω → Ωx, where . The range of the X function is denoted by
.
A discrete random variable is a random variable that can take on finite or countably infinite values.
Suppose we have S ∈ Ωx:

This is the probability that S is the set containing the result.
In the case of random variables, we look at the probability of a random variable having a certain value instead of the probability of obtaining a certain event.
If our sample space is countable, then we have the following:

Suppose we have a die and X is the result after a roll. Then, our sample space for X is Ωx={1, 2, 3, 4, 5, 6}. Assuming this die is fair (unbiased), then we have the following:

When we have a finite number of possible outcomes and each outcome has an equivalent probability assigned to it, such that each outcome is just as likely as any other, we call this a discrete uniform distribution.
Let's say X∼B(n, p). Then, the probability that the value that X takes on is r is as follows:



A lot of the time, we may need to find the expected (average) value of a random variable. We do this using the following formula:

We can also write the preceding equation in the following form:

The following are some of the axioms for :
- If
, then
.
- If
and
, then
.
.
, given that α and β are constants and Xi is not independent.
, which holds for when Xi is independent.
minimizes
over c.
Suppose we have n random variables. Then, their expected value is as follows:

Now that we have a good understanding of the expectation of real-valued random variables, it is time to move on to defining two important concepts—variance and standard variables.